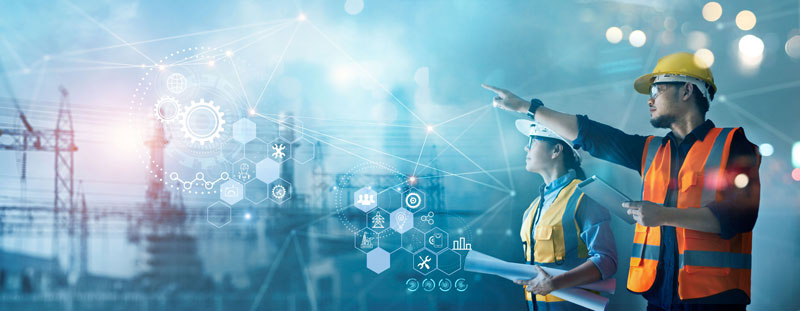
An infrastructure that will support machine learning
- 2 minute read
-
Author: Mobica
The widespread adoption of machine learning (ML) looks set to spark a new industrial revolution, one that could change the face of industry and manufacturing as we know it.
With the potential to rapidly boost performance, enhance efficiencies and improve safety – and, also, tackle growing issues like sustainability and labour shortages – this technology is a genuine game changer.
But to harness its undoubted potential, organisations must first lay the groundwork for success.
ML isn't plug and play
ML is not a turnkey solution. You can’t just plug in a solution and immediately reap the rewards. To leverage the potential benefits, organisations will need to install the right technical infrastructure.
But this is often easier said than done. Not many businesses will want to shut down production lines to rip out old tech and deploy new solutions. So, in most cases, where organisations are working with brownfield sites, machine learning solutions will need to be integrated with legacy systems.
ML is dependent on data, so companies may need to install an array of sensors to monitor multiple factors – such as vibrations, images and streamed video, audio, temperature, humidity, etc. This can mean adding thousands of these sensors within a live industrial environment.
Infrastructure challenges
Often positioned in hard-to-reach locations, these sensors may need to remain in place for perhaps 10-15 years, so thought needs to be given to how they are all going to be powered.
This is not the only challenge which will require careful consideration, however. Once data is harvested, it will need to be transferred for processes. And sending this amount of information around a live production environment can create its own problems – such as the potential interference with existing systems and the risk this poses in terms of security vulnerabilities.
This will likely require companies to invest in a dedicated solution such as a new wireless network or 5G solution. With all this data needing to be stored and processed in the most efficient and cost-effective way, it will also involve the deployment of new Edge and Cloud computing solutions.
Beyond the technological implications
Even with that infrastructure in place, before ML solutions can be effective companies will also need to source expert support to train the initial algorithm. Data analysts will also be needed to check the quality of the information being supplied and continuously retrain the algorithms to maintain the quality of the output.
As ML can bring about significant change to the way organisations operate, business will need to consider the impact on their workforce too. Deployments will need to be accompanied by a change management project, involving education and training programmes.
Other considerations also include what to do if a machine learning solution makes a mistake. This can have serious consequences and, as such, will require businesses to carefully weigh up where they are comfortable deploying these solutions.
Careful consideration
Deploying ML can be complex, and every environment will throw up its own complications. But none of these challenges are insurmountable.
By carefully assessing the potential obstacles and understanding what you can do to overcome them, you can put foundations in place that will allow you to unlock the benefits of machine learning for your organisation.
If you’re looking to take this step, you can prepare yourself and your organisation by reading the latest Mobica Whitepaper, Integrating Machine Learning within Industrial Environments.