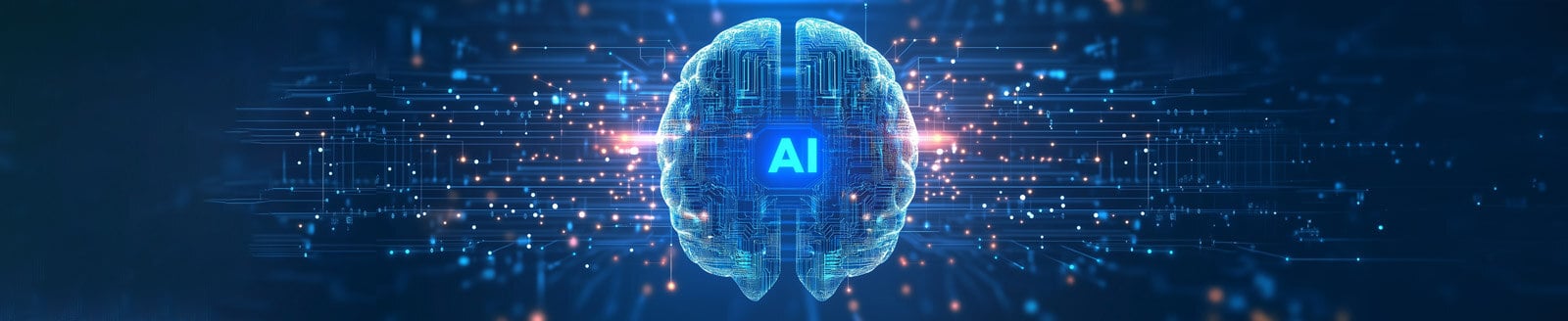
Has the time come to deploy AI at the Edge?
- 2 minute read
-
Author: Mobica
The computing power available on an Edge device is always going to be limited. So you may not think this would be the best place to run a power-hungry AI model. Yet, there are plenty of reasons why organisations would prefer to deploy AI at the Edge, rather than in the Cloud.
While companies want AI to help them roll out technologies such as advanced robotics and industrial automation, they sometimes want these solutions to be free from dependence on Cloud connectivity. They may have concerns over the speed and reliability of the connection, or the possibility that this would open up a new attack vector for hackers. Or it could simply be that they want to minimise their Cloud traffic to keep costs down. There are numerous justifications for Edge AI.
The good news for organisations with those concerns is that a new class of System-on-Chip (SoC) processors is making Edge AI deployment ever more possible. Many of the vendors in this space are now designing chipsets that can be applied to specific use cases too.
For example, Intel is doing work to support computer vision deployments, Qualcomm is helping to improve the capabilities of mobile and wearable devices and Ambarella is advancing what’s possible with video and image processing. We also have Nvidia producing versatile solutions for applications in autonomous vehicles, healthcare, industry and more.
SoC processors that are designed for AI inference, rather than for energy intensive model training, are also helping to make deployments possible on the battery-powered devices commonly used at the Edge.
Stepping to the Edge
Rather than jumping to the Edge, however, any organisation should follow carefully planned steps to ensure their deployment is successful.
For example, Edge AI inference requires models be developed in the Cloud first. Given the availability of scalable computing power, the Cloud is a much better place for developing and training AI models. It’s only when these Cloud models are fully mature that businesses should transition them to the Edge.
On reaching this point, you’ll need to consider all the ways you can keep your data processing to a minimum. This will involve reviewing the specific use case, the capabilities of the selected SoC and their Edge device components, including the cameras and sensors that will be supplying the data.
You’ll then need all the optimisation techniques at your disposal to minimise the size of these data inputs. This will likely involve a fair degree of experimentation to find the lowest level of decision-making accuracy, before quality is compromised too much.
Inference at the Edge will also require the AI model itself to optimise. This will almost certainly involve several transformations, as the model goes through quantisation and simplification processes.
The final step is to address extensibility in order to enable future interoperability with third party systems. This could involve the development of a dedicated API to support the integration of internal and external plugins and the creation of a software development kit to ensure hassle free deployments.
Given the advancements in this field, organisations should now be looking at the potential benefits deploying AI at the Edge rather than in the Cloud. You just need to follow the right steps to get there.
If you want to learn more about how your business can do this, download our new guide Powering AI at the Edge.